Scott Kovaleski, Derek Anderson, Matt Maschmann, Giovanna Guidoboni
Materials Problem
Metasurfaces shape electromagnetic wavefronts to elicit atypical absorption, reflection, or transmission. Metasurfaces consist of geometric unit cells that are fabricated using advanced lithography techniques at dimensions smaller than the wavelength of light to be manipulated (typically sub-microns). The parameter space for metasurface design is enormous and encompasses both material composition and geometric configuration.
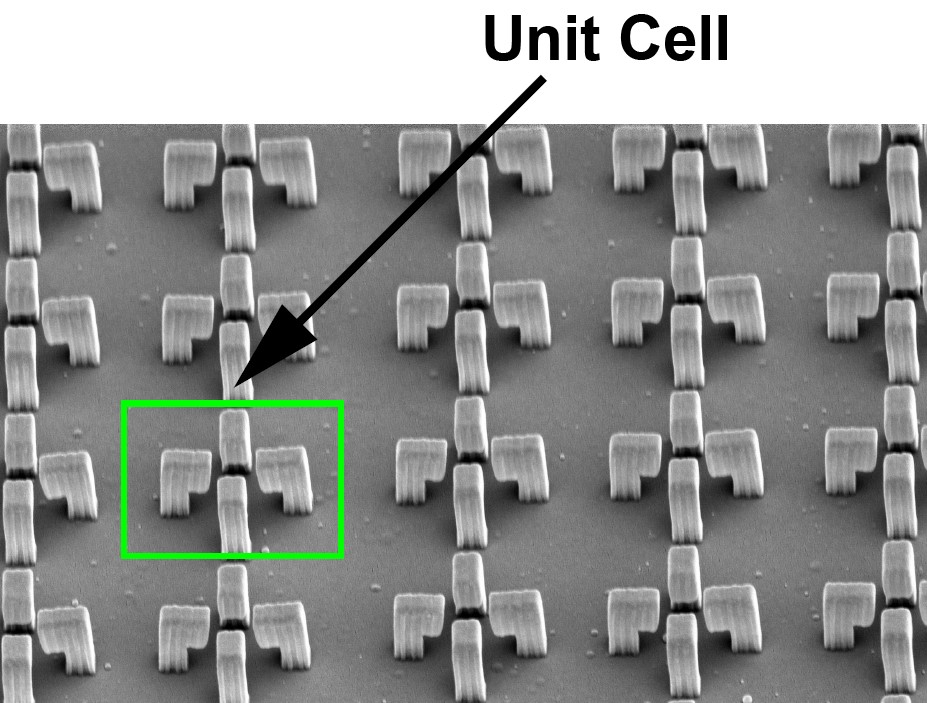
We employ a two-photon lithography (Nanoscribe Quantum X Shape) system to print 3-D metasurfaces with a resolution of approximately 100 nm. Thin film metal and oxide coatings customize the response of printed surfaces. Metasurfaces have demonstrated the ability to produce arbitrary holographic images as well as all-optical neural networks based on layers of designed diffractive surfaces.
Data Science Approach
The number of material and geometric parameters presents a tremendous challenge for metasurface design. To achieve a desired objective, thousands to millions of individual meta-atoms may need to be individually tuned. Meta-atom geometry, morphology, and composition may have been derived from intuition or adapted from larger scale electromagnetic elements, potentially leaving unexplored other meta-atoms that might have advantages in performance or manufacturability. This largely unexplored, high-dimensional design space opens exciting opportunities to use machine learning (ML) techniques for metasurface design. End-to-end optimization connects differentiable wave optics and modern deep learning to simultaneously simulate and optimize diffractive optical elements for deep diffractive neural networks, computational imaging tasks, and real-time photo-realistic holography. Use of inverse design techniques and neural network models will be used to suggest unique metasurface design.
Opportunities for Creativity
Creativity will be essential in exploring how to use ML effectively in the area of metasurface design. For example, how can we reduce the amount of data needed for ML training? What 3-D meta-atom architectures can produce a new exotic response? What are new applications of metasurfaces? Can the process be easily scaled for roll-to-roll processing?